Gamification As a Predictive Tool – From Training To Betting
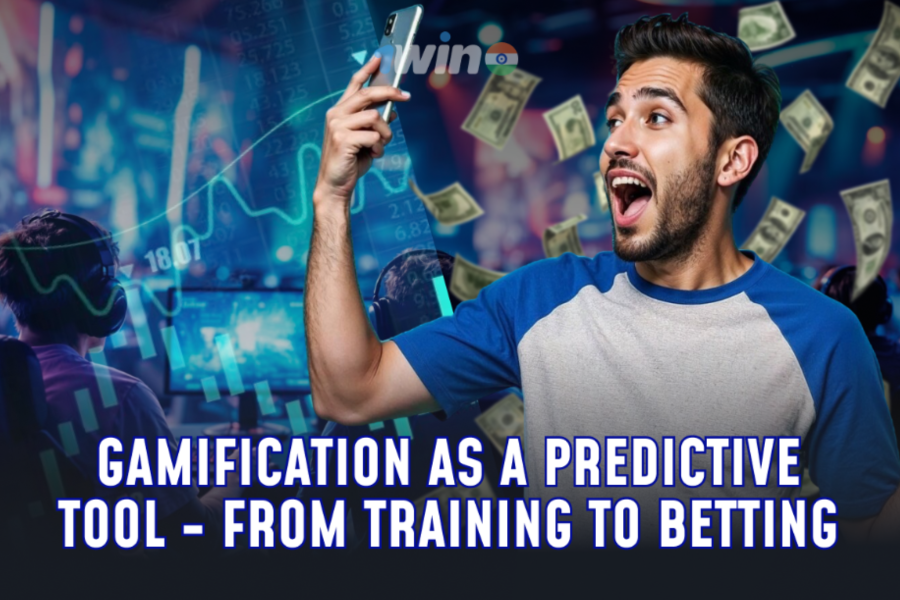
Gamification enhances learning engagement through game-like elements. This concept may have applications in outcome prediction for betting scenarios. This article examines gamification’s potential role in forecasting results objectively.
Understanding Gamification in Learning
Gamification in learning, much like engaging platforms such as 1win online, enhances educational activities by incorporating game design elements. This approach uses points, badges, leaderboards, and challenges to increase student engagement and motivation. The primary objective is to make the learning process more enjoyable and effective.
Key techniques in gamification learning include awarding badges for task completion, creating competitive leaderboards, providing personalized feedback, and enabling real-time progress tracking. These methods tap into human desires for achievement and competition.
Educators can transform learning experiences through gamification, often resulting in increased student participation. However, careful implementation is crucial to avoid overreliance on external rewards. When properly executed, gamification learning can significantly improve educational outcomes.
Definition and Examples
Gamification in education incorporates game elements to enhance learning experiences. This approach utilizes various learning mechanics and gaming mechanics to boost engagement and motivation.
Learning Mechanics | Gaming Mechanics |
Goal setting | Point systems |
Feedback provision | Level progression |
Progress tracking | Badge acquisition |
Personalized paths | Leaderboard rankings |
Collaborative tasks | Virtual rewards |
Types of Data Generated by Gamified Systems
Gamified learning systems produce various data types that provide insights into learner engagement and progress:
- Activity tracking: Measures time spent on tasks, login frequency, and challenge participation. This data reflects overall engagement levels and player behavior.
- Performance metrics: Includes points earned, badges collected, and leaderboard rankings. These indicators demonstrate learning progress and competitive drive.
- Behavioral data: Captures choices made in scenarios, resource management, and peer collaboration. This information reveals decision-making strategies and teamwork preferences, highlighting behavioral patterns.
- Learning analytics: Tracks completion rates, quiz scores, and content interactions. These metrics offer a comprehensive view of knowledge acquisition and skill development.
Connecting Gamification Data to Betting Outcomes
Gamification data analysis may provide insights into betting patterns. Examining player engagement, achievements, and styles could reveal correlations with betting behavior. For instance, a player who consistently chooses high-risk challenges in games might be more likely to place riskier bets. This potential link explores betting psychology, offering a theoretical framework for predicting outcomes. However, it’s crucial to note that this approach remains speculative and does not guarantee accurate betting predictions.
Esports Betting Applications: Training Data Insights
Esports training platforms utilize gamification to collect valuable player data. This information could potentially inform esports betting strategies. Here’s a process to leverage this data:
- Collect performance metrics from training platforms
- Identify trends in player behavior and skill development
- Correlate training data with specific betting markets
- Analyze historical betting patterns in relation to player performance
- Develop and test predictive models
Indirect Applications in Sports Betting
Fantasy sports engagement data provides valuable insights for betting analysis. This information can reveal trends in user behavior and preferences, potentially informing sports betting strategies. In India, where interest in sports betting analysis is growing, this data becomes increasingly relevant.
The table below compares fantasy sports engagement data with traditional sports betting factors:
Fantasy Sports Data | Traditional Betting Factors |
Player selection trends | Team and player performance |
User spending patterns | Odds fluctuations |
In-game engagement metrics | Match statistics |
Social interactions | Public sentiment |
Predictive Modeling Using Gamification Data
Developing prediction models from gamification data involves several key steps:
- Collect and clean gamification data
- Select relevant features for analysis
- Apply learning algorithms to build models
- Train models on historical data
- Validate models using performance metrics
- Deploy models for real-time predictions
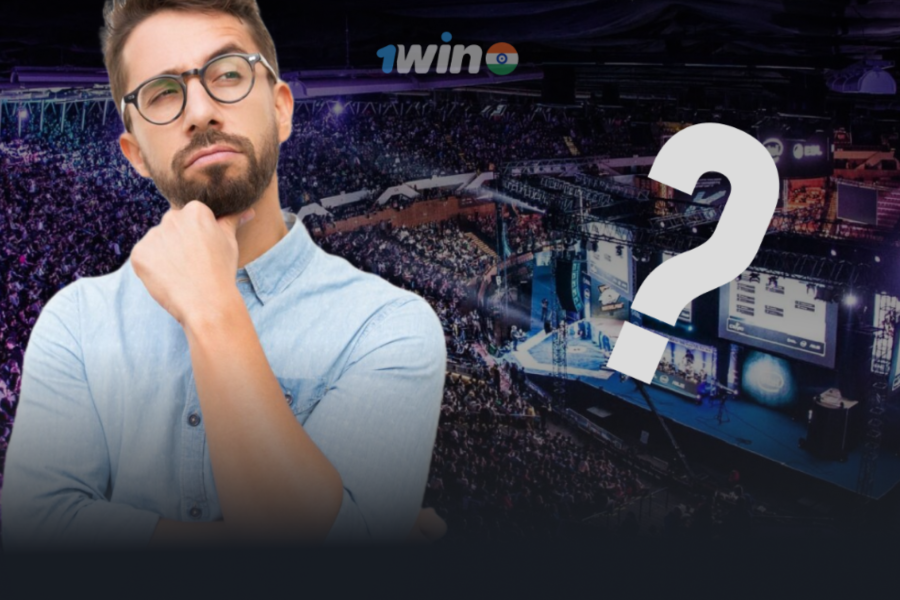
Challenges in Using Gamification Data for Betting Predictions
Utilizing gamification data for betting predictions presents several complexities. Esports metrics from training platforms can identify skilled players but may not directly translate to real-world performance. Fantasy sports data offers insights into fan sentiment but lacks reliability for actual outcome prediction. Developing algorithms to integrate this information with other factors requires advanced data science skills. While gamification data may provide some advantages, it is crucial to recognize its limitations in predicting final results accurately.
Data Privacy and Ethical Considerations
Using gamification data for betting purposes raises important ethical questions. Responsible gambling education is crucial when implementing predictive technologies in betting. Key ethical considerations include:
- Obtaining explicit user consent for data collection
- Anonymizing player information to protect privacy
- Maintaining transparency about data usage practices
- Limiting access to prevent misuse in betting algorithms
- Implementing safeguards against addiction triggers
Complexity of Data Analysis
Analyzing complex gamification data for betting prediction models presents significant challenges. Effective prediction models require processing vast amounts of information from gamified systems, including player actions, progress, and preferences. Selecting appropriate inputs and machine learning techniques is crucial for accurate forecasts. This process involves training models on historical data and continuous monitoring.
The table below compares data analysis requirements:
Aspect | Simple Cognitive Gaming | Complex Betting Prediction Models |
Data Volume | Limited | Extensive |
Variables | Few, game-specific | Numerous, including external factors |
Analysis Depth | Basic statistics | Advanced machine learning |
Update Frequency | Infrequent | Continuous |
Limitations in Predictive Accuracy
Gamification data for betting predictions has inherent constraints. Several factors can impact the accuracy of these models:
- Sample bias: Gamified users may not represent all bettors
- Lack of real stakes: Virtual behavior differs from actual betting
- External variables: Injuries, weather, and other unpredictable elements
- Overfitting risk: Models may perform poorly on new data
- Evolving trends: Betting patterns change over time
Future Prospects and Research Needs
Gamification data presents promising applications for enhancing betting outcome prediction. By analyzing user behavior in gamified environments, researchers can develop more sophisticated prediction models. These models could incorporate insights from game psychology and decision-making theory to improve accuracy.
Areas for further research include:
Research Area | Questions to Explore |
Data Integration | How can gamification data be effectively incorporated into existing prediction models? |
Accuracy Assessment | What is the impact of gamification-derived insights on predictive accuracy? |
Ethical Considerations | How can we ensure the responsible use of gamification data in betting predictions? |
You can also read: A guide on betting for Indians